Understandable modelling with modular building blocks
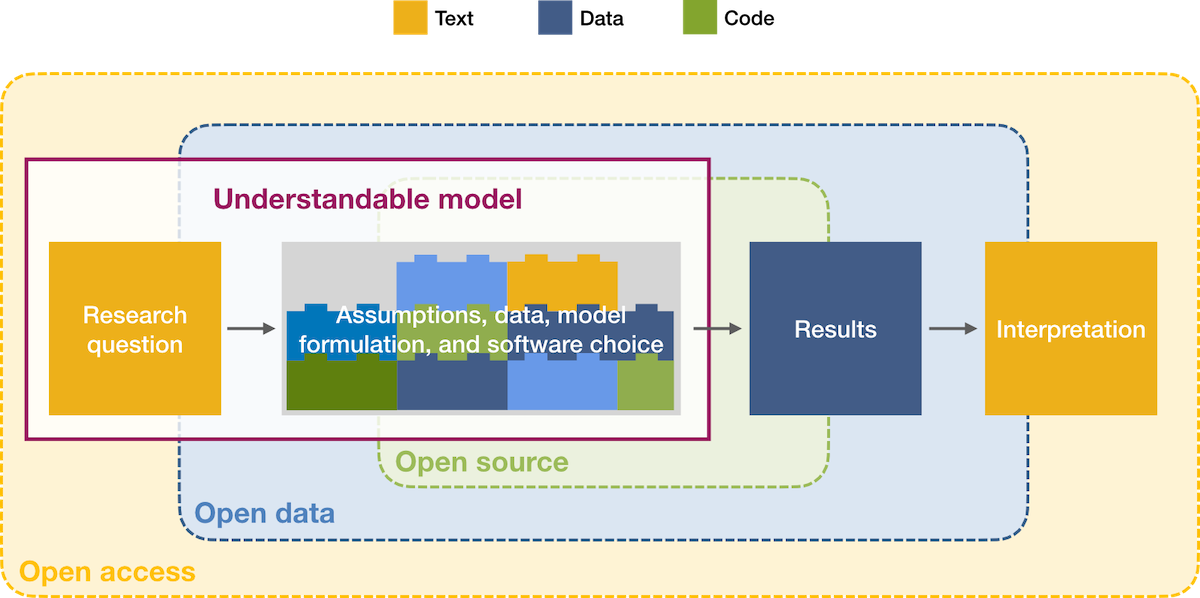
Energy system models are assumption-based representations of complex systems, making validation impractical. Post-normal science suggests instead a focus on communicating values and uncertainties. Open energy modelling can support this by breaking models into manageable parts. Collaboration on these components (whether code or data) would simplify and accelerate model development, while improving accessibility and understandability for users. → Pfenninger (2024), Progress in Energy.
Read more...